Executive summary:
Cash flow forecasting is becoming increasingly important in times of economic uncertainty. However, many companies face challenges because their forecasting processes are often manual, complex and prone to errors. Artificial intelligence can help by analysing large amounts of data in real time to identify trends and patterns, enabling more accurate cash flow forecasts. This reduces manual effort, improves planning quality and supports informed financial decisions. In this article, we discuss the advantages, prerequisites and challenges of AI-supported cash flow forecasting and walk you through its practical implementation.
Cash flow forecasting is a fundamental part of corporate financial planning. Given the current interest rate environment and ongoing concerns about a possible economic downturn, liquidity management is becoming increasingly important and, according to surveys such as the EACT Treasury Survey and the Deloitte Global Treasury Survey, is increasingly becoming the focus of financial managers. Accurate planning helps to make informed financial management decisions and to identify liquidity shortages at an early stage, even in volatile market conditions, so that proactive measures can be taken in good time.
Despite its strategic relevance, cash flow forecasting is not usually one of the preferred tasks of treasurers, as it often involves a high level of manual effort, complex data integration and uncertainties. Almost 40% consider their forecasting skills to be below average and in need of improvement.
Artificial intelligence provides a solution here. Machine learning can be used to automate time-consuming manual processes and increase planning accuracy and frequency. AI algorithms can analyse large amounts of data in real time and deliver more accurate cash flow forecasts, enabling informed decisions to be made.
This article is based on a conversation with Hubert Rappold, Senior Treasury Expert at Nomentia, and a paper he co-authored on AI in cash flow forecasting. Below, we discuss what exactly AI-based cash flow forecasting means, what advantages and challenges it offers, and how implementing it with a software can look like
About our expert
Hubert Rappold is Senior Treasury Expert at Nomentia. After 10 years as a partner at Schwabe, Ley & Greiner, he founded TIPCO, where he was Co-CEO for 10 years. Since Nomentia acquired TIPCO in 2021, he has played a key role in building the Nomentia brand in German-speaking countries in various roles.
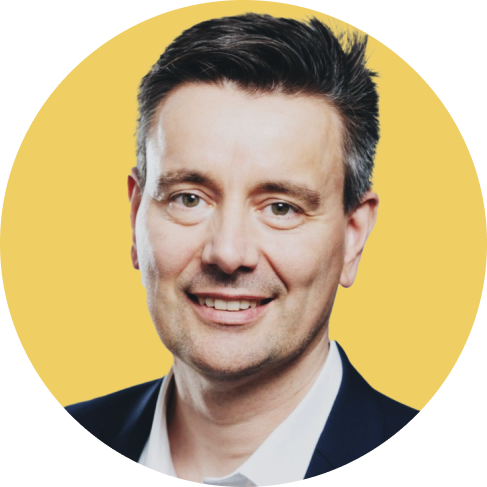
Why AI in cash flow forecasting?
Whether using Excel spreadsheets or software, the importance of effective cash flow forecasting to ensure solvency and avoid liquidity shortages is undeniable. While many companies still plan manually using Excel spreadsheets, others are already using centralised software solutions that automate data collection and reporting. The next step in optimising liquidity management is to support cash flow forecasting with artificial intelligence. Compared to conventional methods, this not only means significantly less manual work, but also an increase in planning accuracy. This is because algorithms also recognise trends and patterns that are difficult to detect with the naked eye. In addition, a large number of internal and external influencing factors can be included in the liquidity forecast, which is virtually impossible to do manually. This leads to higher planning quality with significantly reduced effort. "Once the AI is set up, forecasts and and forecassting methods are automatically updated based on new data and historical analyses", explains Hubert Rappold, Senior Treasury Expert at Nomentia.
By automating the process of cash flow forecasting, treasury teams can save a lot of time and hence also increase the frequency of planning. They can recalculate the forecast at any time, for example if circumstances have changed slightly or other influencing factors need to be taken into account. Another advantage of automation is that the calculations become more accurate each time more actual data is added. Nomentia customers report an accuracy rate of up to 95 percent for long-term planning over a time horizon of approximately six months.
How does AI work in cash flow forecasting?
The type of AI used for cash flow forecasting falls under the umbrella of 'predictive analytics'. It uses methods from the fields of classical statistics and machine learning, which differ significantly from the typical idea of uncontrollable generative artificial intelligence. In concrete terms, predictive analytics in cash flow forecasting consists of various algorithms, also known as models or methods in statistics, which identify patterns in historical payment data and use them to calculate forecasts.
In software, this works as follows: In an initial testing phase, various algorithms are applied to historical training data. Depending on the type of data, certain algorithms are more suitable than others. The different forecasts generated are then compared with the actual data to determine their accuracy and select the best possible method for future forecasts. Even after the testing phase, the forecasts are regularly compared with actual cash flows to further improve accuracy.
The entire AI planning process is thus based on a defined database and statistical models that are programmed into the system. This means that sensitive cash flow data is processed in the secure environment of the solution and is not passed on to external providers.
Who can benefit from AI in cash flow forecasting?
The possibility of using machine learning for cash flow forecasting depends primarily on the data available. ‘In theory, AI-based cash flow forecasting is possible for any company as long as the data pool is large enough and centralised,’ explains Hubert Rappold, Senior Treasury Expert at Nomentia. However, companies that have already categorised their actual cash flows in the past will find it easier.
However, pattern recognition depends not only on the volume of data, but also on how consistent the cash flows are. The process is therefore particularly suitable for companies in the B2C sector or subsidiaries with a broad customer base whose cash flows are not dominated by large individual payments, as useful patterns are easier to identify than in smaller subsidiaries with large projects in the B2B sector, for example. However, the process can also be advantageous for the latter. Even if the data may need to be carefully checked and adjusted and some manual planning is still carried out in parallel, it still offers the advantage that influencing factors can be better identified and are digitally documented rather than isolated in one single treasurer's head.
In terms of the planning horizon, AI is suitable for both long-term planning and short-term planning. In long-term planning, AI supports pattern recognition and the creation of various scenarios based on external influences, and can significantly increase planning accuracy. However, the use of AI is also advantageous for short-term 13-week planning or even 14-day planning based on open items, as it saves a lot of manual work and increases the planning frequency. In addition, the ability to present different liquidity scenarios facilitates communication with external partners such as investors and banks. The increased transparency creates trust in this situation and can strengthen the support of partners.
5 steps to implementing AI-powered cash flow forecasting
To be able to leverage AI for more efficient and accurate cash flow forecasting, the following five steps are necessary:
- Review requirements
- Objectives and prerequisites
- Determining and cleaning historical data
- Calibration and validation of methods and quality measures
- Transfer to regular operation and ongoing monitoring
1. Review requirements
A stable database of actual data is essential for the successful use of AI in cash flow forecasting. ‘In order to make accurate forecasts, historical cash flow data covering at least three to four years must be available so that patterns and seasonal trends can be identified,’ explains Hubert Rappold.
The data must also be classified and complete. This means that the data must clearly show whether it relates to operating cash flows relevant for the analysis, such as customer receipts and supplier payments, or special cash flows that should be excluded from planning, such as financing and dividends. The cash flows should also not show any significant distortions or gaps. For example, it is difficult to identify patterns for a stable liquidity forecast from fluctuating cash flows following a company merger.
Once the data is available, the question of expertise within the team arises. Many treasury departments lack the statistical, mathematical and programming expertise required for such a project, especially in small and medium-sized companies that do not employ their own data scientists. External consulting or a suitable software solution is therefore usually required for implementation. Although special software is not a mandatory requirement, it does make the process considerably easier. Alternatively, simple in-house solutions using Excel and freely available calculation engines can be used, for example for getting started or prototyping. However, these quickly reach their limits when it comes to large amounts of data, and the results are often not traceable. Caution is also advised with online platforms that offer automated forecasts based on uploaded time series. These ‘black box’ systems do not disclose their calculation methods, require large amounts of data and should also be viewed critically for data protection reasons.
The simplest and safest option therefore remains the use of a solution integrated into the ERP or TMS system. Treasury management systems such as Nomentia offer the possibility of creating forecasts based on various algorithms and historical data in the system. The providers have the necessary expertise and often offer the option of an inexpensive proof of concept before the actual project is launched.
2. Objectives and prerequisites
Once all prerequisites have been checked and the necessary decisions have been made, you can begin setting objectives and planning the project.
As with conventional cash flow forecasting, the company-specific planning structure and planning principles must first be established. This includes questions such as: Should the planning be direct or indirect? How often should planning be carried out and over what time horizon – long, medium or short term? Is there any external macroeconomic data that is relevant for planning? Is there a need for currency-differentiated cash flow forecasting? And who is responsible for providing and validating the data, as well as for quality assurance?
The first AI-specific question you should ask yourself is how extensive your cash flow forecasting with AI should be and what you expect to achieve. Do you want to supplement existing planning or replace and automate it completely? Do you want to start with a proof-of-concept phase involving just a handful of representative group companies, or go all in right away? Also, determine which planning categories you want to include and which you want to exclude. Regular, operational cash flows such as accounts receivable and accounts payable are particularly suitable for automated planning, while irregular, high cash flows such as those from investments are better planned manually.
Planning the AI project also involves deciding whether the project will be handled in-house or whether external support is needed. Some companies already have an AI project department that can be involved in the project. If this is not the case, external consultants can be brought in for data analysis and the selection of AI models, or software can be purchased.
The decision on whether external support is needed also has an impact on budgeting. When it comes to planning the budget, it is difficult to give concrete figures. Factors influencing the budget include the time required to determine the actual data, the scope of the project – i.e. how many group companies and planning categories are to be included in the planning – the need for external consultants, and the one-off and ongoing costs associated with a potential software purchase.
3. Determining and cleaning historical data
The most important step in AI-supported cash flow forecasting is determining and cleaning historical data, which accounts for around 80 percent of the total workload. If you start with a proof of concept, it is advisable to begin with two or three representative business units so that you can then determine the extent to which it is worthwhile rolling out the concept across the entire company. As already mentioned, the data must be classified and complete in order to enable high-quality planning. ‘It is particularly important that historical data is classified correctly so that it can be assigned to the correct planning category and does not distort the planning results,’ says Rappold. For this reason, it is ideal if the data can be derived from the ERP system using transaction chain analysis, as this already provides the details required for categorising payment data. In a heterogeneous ERP landscape or when working with ERP systems that do not classify transactions, transaction chain analysis is often not possible and a second option must be used, where data is taken from bank statements. With this method, payment references can provide information about the category of the payment stream, but the level of detail is much more limited compared to transaction chain analysis. ‘When determining data from bank statements, we can correctly map and classify up to 90% of payments, but the rest has to be added manually – a huge amount of work that is not always worthwhile,’ explains Rappold.
Robust forecasts can only be made from reliable and correctly categorised data. That is why the data must first be cleaned and analysed after extraction. This involves checking it for completeness and outliers, i.e. high cash flows that do not correspond to normal business activity. If data is missing, for example if an entire month or week is missing, the gaps should be filled with average values so that the forecast is not distorted. Outliers can be automatically identified using box plot models and removed if necessary.
Preparing the data basis also includes determining the factors that influence cash flow development. These range from general, cross-industry factors such as holiday effects, seasonality and trends to individual, company-specific influencing factors such as fixed payment dates, production planning or known outlier events. It is also possible that not many individual factors are known at the beginning. However, these can be identified and adjusted over time the longer the AI-planning is in use, making the planned values more accurate. External data such as inflation, economic trends or wage developments can also be included in the forecast if they are relevant to the company. For example, OECD price indices can be incorporated into planning to take price fluctuations into account, provided that previous planning has revealed a correlation between the cash flow data and the index.
4. Calibration and validation of methods and quality measures
Once a suitable database and relevant influencing factors are available, processing the data is automated. The system automatically selects suitable algorithms. Depending on the group company or planning category, different models can be used and adapted to changing business conditions. Which method achieves the best results varies from case to case. Frequently used models include regression, neural networks and time series analysis.
For the final selection, a test plan is carried out with each model from the preliminary selection. At least two years of historical cash flow data serve as a training basis, while the forecasts are compared with the actual values of the third year. The algorithm with the best results is used for future planning. This process is largely automated in software. Manual adjustments are possible, but only necessary if exceptional events such as an economic crisis distort the data.
In order to ensure the quality of the forecast, so-called quality measures are used. These provide information about the accuracy of the forecast by measuring how much the predicted values deviate from the actual data. There are also different types of quality measures – the most common of which is the RSME, which calculates the root mean square error. Regardless of which quality measure is used to select the models, it is important to check the quality measures regularly. This is because the quality measures are also used to continuously assess whether the selected method is still the best or whether a different algorithm would achieve better results due to changed conditions.
5. Transfer to regular operation and ongoing monitoring
In some cases, the test phase reveals that the quality of the data is not yet sufficient to produce an accurate forecast, or that the effort required to clean and adjust the data is greater than for manual planning. However, if good results can be achieved from the test data in the proof-of-concept phase, AI-based cash flow forecasting can be extended to the rest of the business and incorporated into regular operations.
At this point, it should be noted that automatic planning does not have to completely replace manual planning, nor can it always do so. Ultimately, responsibility for cash flows always remains with the planning units and cannot be replaced by automatically generated forecasts. Many companies view the figures calculated using AI as suggested values for support and base further manual adjustments on them. This decision also depends on the various planning categories. For example, predictions about salaries are usually taken over completely by the system due to their high accuracy, while in categories that are often dominated by exceptions, the calculated data often serve as guidance for additional manual planning. However, even if manual intervention is still required for certain categories, the support provided by the generated forecasts represents an enormous time saving. In addition, if the software provider supplies this information, the estimation results and observations behind the planning data can also be extremely valuable in identifying forecasting errors and determining previously unknown influencing factors.
What's more, while the first run requires more attention and manual adjustments, the forecasts become more accurate over time as more actual data is added. This is because the algorithms are programmed to automatically reconcile the forecasts with the actual data as it arrives, resulting in continuous improvement.
Frequently asked questions about AI in cash flow forecasting
What are the prerequisites for AI-based cash flow forecasting?
The key to AI-supported cash flow forecasting is the data base. This must go back at least three years and contain sufficient details on historical cash flows so that they can be recognised by the algorithm and the cash movements can be assigned to the correct planning category. In order to create accurate forecasts with AI, the relevant expertise is also required. If this knowledge is not available within your team, you will need external consultants or a software solution such as Nomentia Cash Flow Forecasting.
Our experts will be happy to advise you.How much data history do I need for AI to make sense?
Where does the historical cash flow data for the forecast come from?
However, especially in a heterogeneous ERP landscape or when working with ERP systems that do not classify transactions, document chain analysis is often not feasible.
In such cases, a secondary approach must be used, where the data is taken from bank statements.
With this method, payment references can provide some insight into the category of the cash flow, but the level of detail is significantly more limited compared to document chain analysis.
Can AI recognise the payment behaviour of individual creditors, or is this done on a general basis?
How do you select the right models for forecasting?
Different models can yield very different results on the same historical data, depending on the data itself. That’s why it’s crucial to select models based on accuracy, speed, and transparency. Nomentia automatically preselects suitable models based on your data and requirements, but users can also add or exclude models manually. The reasons why a model has been chosen - like for example assumptions like seasonality or holiday effects - are clearly outlined in a report. The definite best model is chosen by running the forecast with the preselected models and comparing the results against actual historical data.
How to prevent "overfitting" of the models?
How long does it take to calculate a forecast with AI?
Does the use of AI for cash flow forecasting pose a data protection risk?
The type of AI used in cash flow forecasting – also known as predictive analytics – is based on selected historical payment data and algorithms that recognise patterns in this data. If AI-based planning is carried out using a software solution, the algorithms are pre-programmed in the system and the liquidity forecasts are calculated within the system itself. Sensitive cash flow data is therefore processed in the secure environment of the solution and is not passed on to external third-party providers.
Find out more about the security measures at Nomentia.How transparent are forecasts generated using AI?
Some providers of AI-based cash flow forecasting, including Nomentia, offer graphical representations that break down the factors included in the forecasts. This creates transparency about how the forecasts were calculated and makes them more transparent. Nomentia also provides a detailed report for each forecast on the internal and external factors taken into account, which can highlight observations and trends that a treasurer might not be able to identify with the naked eye, thus ensuring a deeper understanding of the various influencing factors.
Contact us to learn more about additional features.AI cash flow forecasting with Nomentia
Whether for complete automation or to support manual planning, predictive analytics takes your cash flow forecasting to the next level. Once set up, AI-powered methods optimise liquidity management through more accurate forecasts, deeper insights into cash flow drivers and less manual effort.
With Nomentia, you receive comprehensive support from our specialised data scientists – from the initial analysis to ongoing optimisation. We accompany you through all phases: from a non-binding proof of concept to data analysis and cleansing to calibration, validation and generation of the first automatic forecast. Even after implementation, we are at your side for continuous improvement.